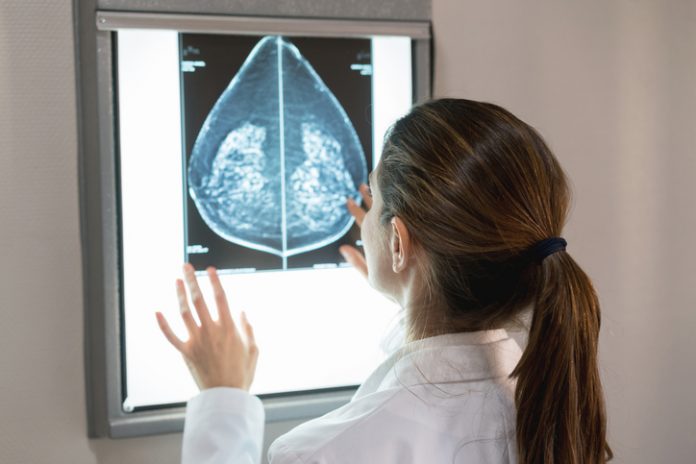
In one of the largest studies of its kind, researchers have used radiomics analysis to identify six distinct breast tissue patterns, beyond breast density, that are associated with breast cancer risk.
“We expect these phenotypes to improve future risk prediction to better identify women at high risk for breast cancer for risk-reduction strategies and tailored screening, as well as women at low risk for breast cancer who may be able to extend screening intervals,” wrote Stacey Winham, from the Department of Quantitative Health Sciences at the Mayo Clinic in Rochester, and co-authors in Radiology.
They explained that although breast density is a known risk factor for breast cancer and false-negative findings on mammogram, women with similar proportions of breast density can have substantial heterogeneity in their tissue patterns.
“Unlike breast density, which is a global image measure, mammographic texture reflects the spatial distribution of the more subtle, localized complexity of breast tissue on the mammogram,” co-senior author Celine Vachon, also from the Mayo Clinic, told Inside Precision Medicine. “We hypothesize that the intrinsic heterogeneity of the structure and texture on a mammogram more accurately reflects the underlying predisposition of the breast to develop breast cancer and provides complementary information to breast density for breast cancer risk.”
To test this hypothesis, the researchers used radiomics to automatically identify and extract quantitative features from more than 30,000 digital mammograms obtained at three diverse breast screening practices.
“We systematically sampled almost 400 different mammographic features at various points throughout the entire mammogram image to get a comprehensive representation of texture and structure of breast tissue,” said Vachon. “We then used this information to develop six parenchymal phenotypes, or breast tissue patterns, that represent this variation.”
The phenotypes were developed using two complementary statistical approaches: hierarchical clustering and principal component (PC) analysis.
“The phenotype ‘clusters’ represent mutually exclusive sub-groups or categories of patients, whereas the PCs represent continuous scores based on a linear combination of the radiomic features,” Vachon explained. “The discrete subgroups defined through hierarchical clustering may improve interpretability and clinical utility by grouping patients into categories, whereas the continuous PCs reduce the high dimensionality of the radiomic features into a quantitative score and improve power for testing associations with breast cancer outcomes.”
These six phenotypes were then validated on mammograms from over 1,055 women with invasive breast cancer and 2,764 women without breast cancer.
The researchers reported that the six clusters and six quantitative PCs were significantly associated with a higher risk for invasive breast cancer after adjustment for age, body mass index, and Breast Imaging Reporting and Data System (BI-RADS) breast density.
Although the two methods provide similar information, the PCs allowed for greater power when examining the associations with breast cancer endpoints, Vachon noted.
Specifically, adding the PC information to age, BMI, and BI-RADS breast density significantly improved the accuracy for predicting overall breast cancer in Black women from 63% to 68%. The improvement from 60% to 61% was not significant for White women. However, the researchers noted that the odds for predicting cancer with PCs did not differ significantly between Black and White women.
Adding the PC phenotypes also significantly improved the accuracy, from 66% to 73%, for discriminating false negative findings, and from 68% to 77% for predicting interval cancers.
This suggests that “the PCs may be incorporated into risk prediction of [false-negative] findings to identify women at highest risk of missed and/or fast-growing tumors, which may inform supplemental screening recommendations,” Winham et al remarked.
“Our next steps are to understand whether these phenotypes contribute to known risk factors for breast cancer and established risk models for breast cancer,” said Vachon. “Our hope is that this information can be used in addition to current risk models to better stratify women at high and low risk.
She added: “We plan to extend their investigations to larger groups of women in the U.S. population, especially examining 3D mammograms, and combining these radiomic risk factors with genetic and other lifestyle factors to improve our ability to define who is (and is not) at increased risk of invasive breast cancer.”